GUIDE
The mobile marketer’s essential guide to incrementality analysis
In a galaxy far, far away, marketers are relying solely on last-touch attribution, relishing the abundance of real-time, device-level data available to them. In our galaxy, we live in a very different reality. Increasingly strict data privacy regulations, economic uncertainty, and the rise of user acquisition (UA) costs, require marketers—more than ever—to be more effective at adapting strategies in real-time–optimizing marketing efforts swiftly for maximum impact and growth.
It’s a new era, which requires adding new, innovative solutions on top of the tried and trusted last-touch attribution. This move away from granular user-level data, toward incorporating more aggregated methodologies of campaign measurement, can complicate a marketer’s ability to deeply understand the true impact of their efforts. This is where incrementality analysis comes into play.
Incrementality definition
What is incrementality in marketing?
Incrementality in marketing is a way of measuring a marketing action’s effect on a specific target key performance indicator (KPI) to determine whether the campaign is providing incremental lift, having a negative impact, such as cannibalizing organics, or making no impact.
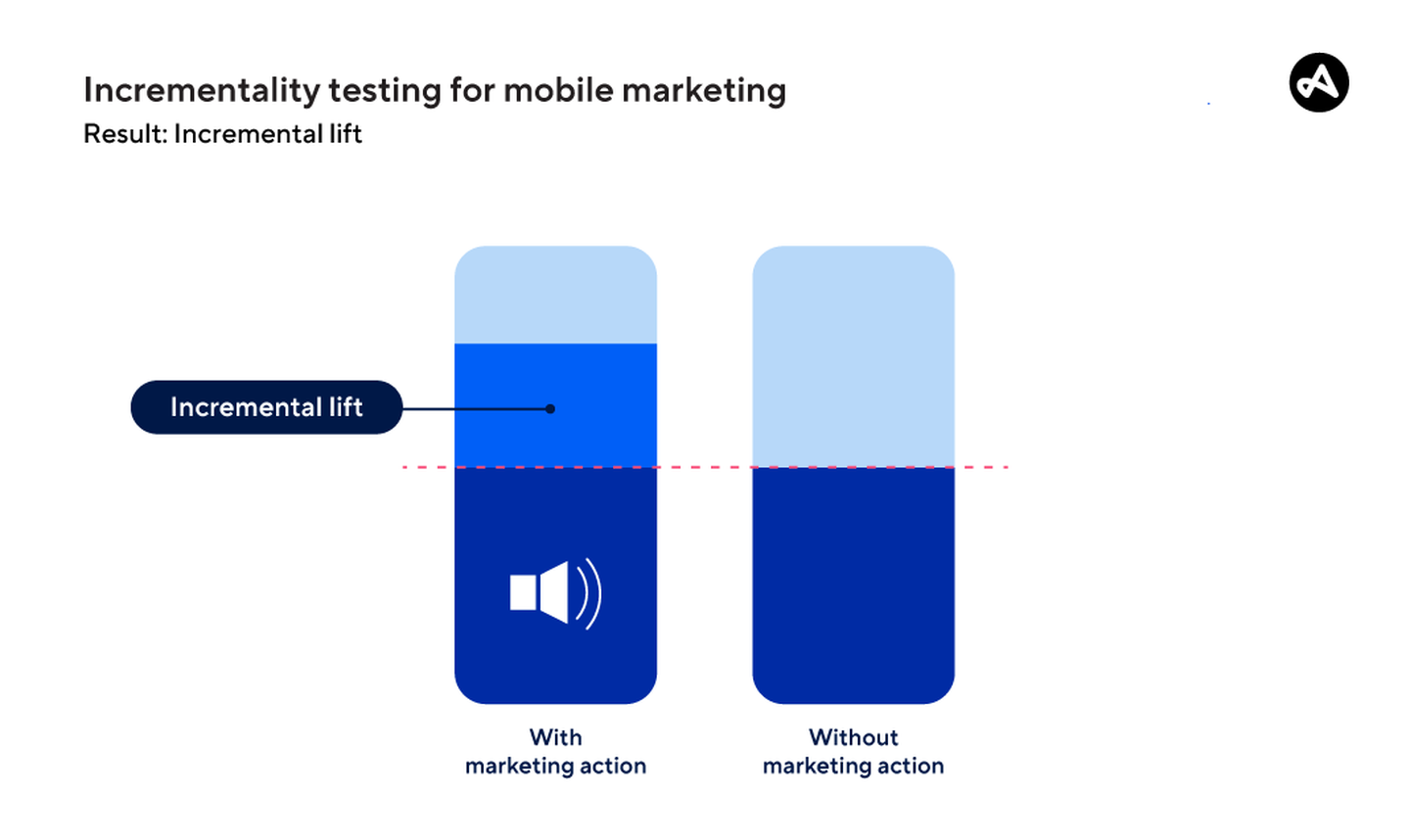
With incrementality analysis, marketers can confidently gauge whether or not a particular campaign positively affected a specific outcome, such as increased sales. It can also help assess whether the campaign was necessary, or if the company would have achieved the same results if the campaign had never happened.
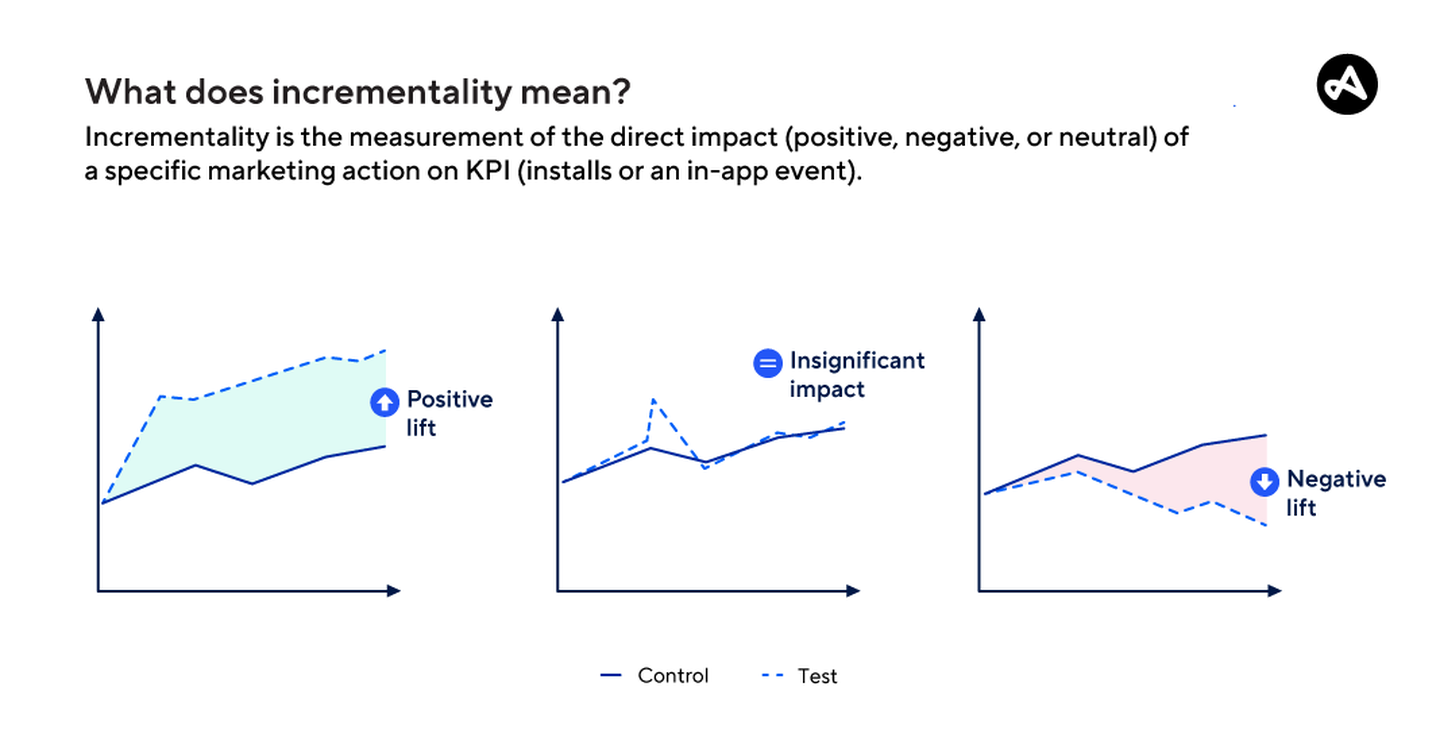
If done right, incrementality testing can answer the question, “What would have happened if I had not done X?” with high precision.
Your incrementality test results will tell you the exact, direct impact of an individual marketing effort on a given KPI. That might look a little something like this:
Question | KPI | Incrementality test result | Answer |
---|---|---|---|
What would have happened if I had not run this campaign? | Installs | Positive lift | My app would have received X% less installs without the campaign. |
What would have happened if I had not increased my budget by X? | Event: First session | Negative lift | My app’s users would have had significantly more first sessions without the budget increase. |
What would have happened if I had not used this new advertising channel? | Installs | Insignificant impact | My app would have received the same number of installs without this campaign. |
However, these outcomes can result in more complex insights, as well. Take a look at a couple of examples.
Question | KPI | Incrementality test result | Answer |
---|---|---|---|
What would have happened if I had not decreased my ad spend? | Event: Game level completion | No significant lift on Android, negative lift on iOS | Roughly the same number of users would have completed level 5 on Android, while X% more users would have completed level 5 on iOS. |
What would have happened if I had not run this campaign on Facebook and ASA?* | Installs | No significant impact with Facebook, positive lift with ASA | If I had not run this campaign on Facebook, my app’s installs would have looked about the same. But if I had not run this campaign with ASA, my app would’ve had X% fewer installs. |
*At this time, only one network can be tested at a time with Adjust InSight.
Adjust and incrementality
How to measure incrementality
Incrementality analysis can be done in a few different ways. At its core, incrementality works by leveraging causal data science models and control groups (more on this below), which tells us what would have happened in the absence of a marketing action. In other words, no more guessing, “What would happen if I doubled my budget with network A, or stopped my search campaigns entirely?”
Traditionally, the approach was to segment a target audience into a control group and a test group. The test group was exposed to the marketing campaign, and the results were compared against the control group. In essence, A/B testing.
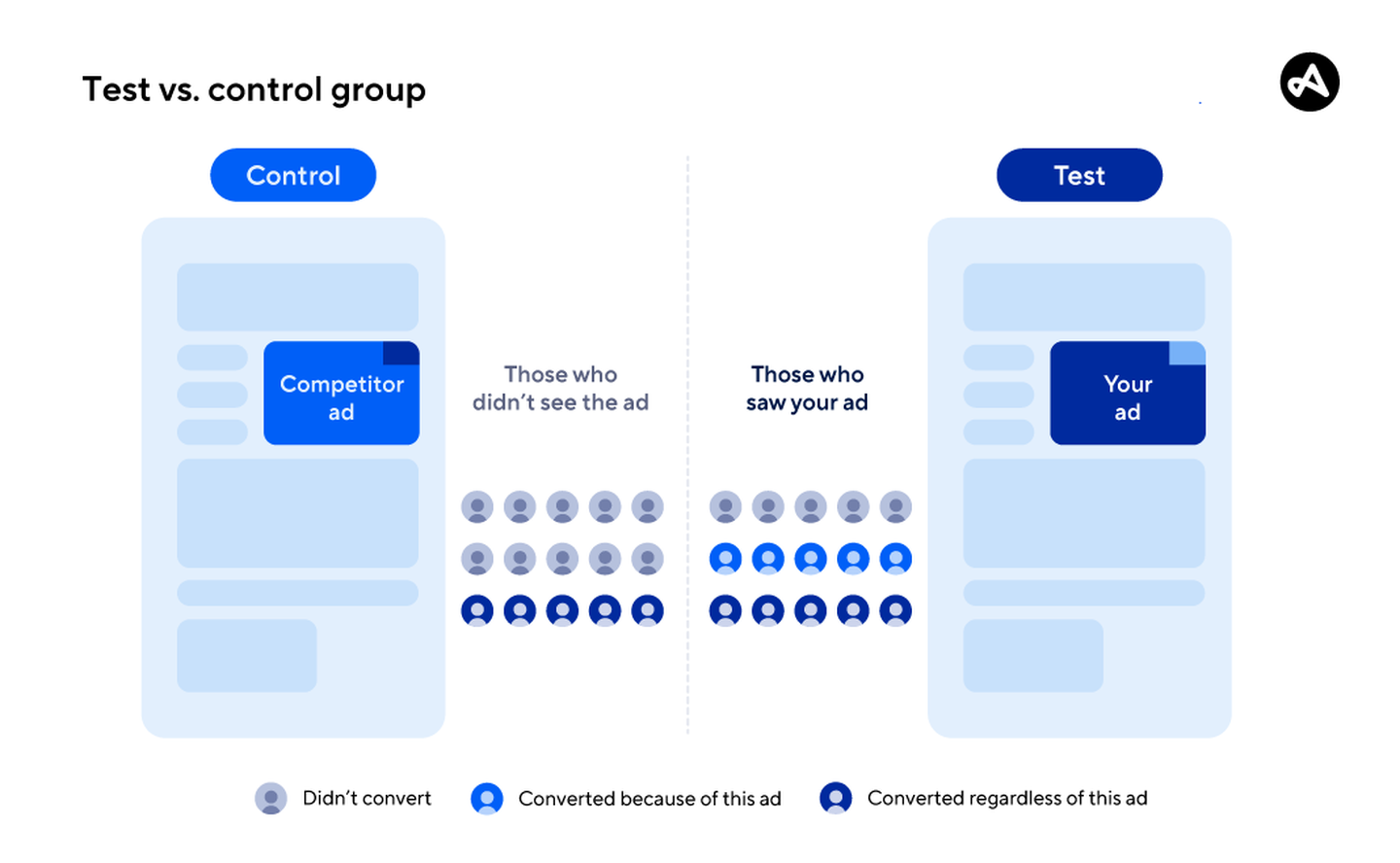
This approach is not very reliable for three key reasons:
- It can result in biased or inconclusive results.
- It’s very challenging to guarantee data sanitization because the control group might be exposed to other campaigns running at the same time.
- It takes a lot of effort to create control groups that are large enough and similar enough to the test group to minimize variables as much as possible for high accuracy.
InSight: The Adjust approach to incrementality
Adjust strives to offer data and insights that are as accurate as they are reliable, in a privacy-centric manner. So, we tweaked this traditional take and molded it into something truly future-proof for the modern marketer. Adjust's approach to incrementality analysis, through our InSight solution, has two key differences. InSight:
- Uses aggregated historical attribution and ad spend data, combined with data observed from significant external factors, to create a synthetic control group.
- Leverages a machine learning model to make the comparison between the test and control data groups with 95% accuracy.
For each analysis, the synthetic control group will closely correlate with the app in question in both app metrics and user behavior. This also ensures that no marketing campaign needs to be paused to find true incremental lift.

As a result, marketers will have the ability to understand if a campaign or channel is driving incremental lift for their app or determine if they are hindering their growth by cannibalizing organic traffic. This enables them to adapt swiftly in real-time and optimize strategies for maximum impact and brand growth.

Incrementality analysis use cases
There are many ways a few specific ways Adjust’s method of incrementality measurement can be leveraged. Here are some of the most common ones.
- Seasonality testing**: Validate how effective seasonality is to, for example, increase budget around big holidays.
- Advertising channel discovery: Validate the efficacy of new advertising channels when it comes to certain events.
- Platform-based optimization: Understand if the channel in question yields better results for iOS or Android.
- Ad fatigue detection: Verify if a campaign has reached ad fatigue.
**Seasonality testing is not available for all tests due to data availability.
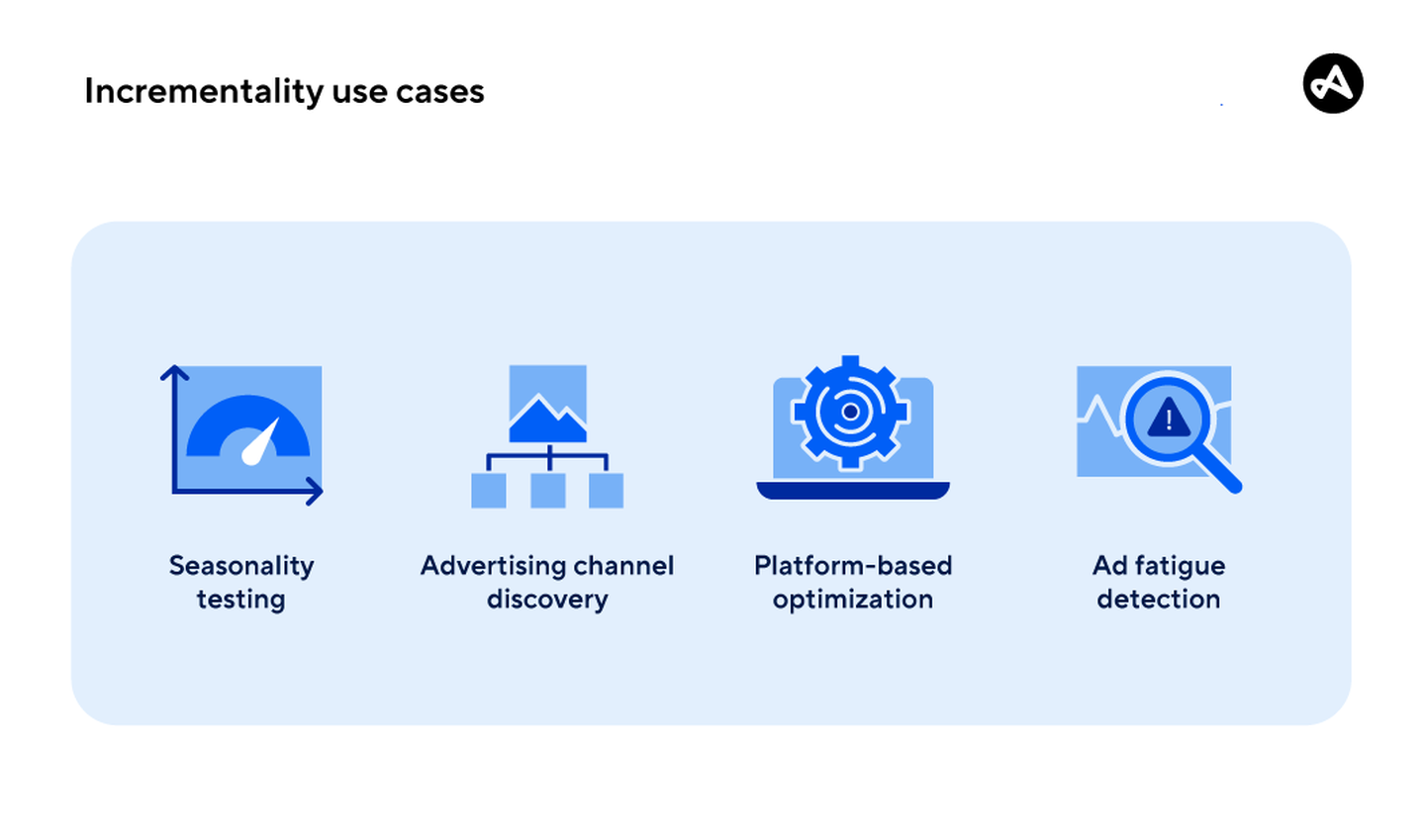
Examples
Understanding the ins and outs of InSight for incrementality analysis
Let’s take a look at how this actually works, in practice.
- First, a marketer identifies which marketing action, campaign, country, and timeframe the analysis should be for. Then, they identify if the incrementality test will be analyzing performance based on installs or events.
Example: I want to validate the performance of network A in the U.S. when it comes to converting users to make a first purchase during the week before “first day of school”.
2. Then, the testing period is chosen. There must be enough historical data to calibrate the machine learning models accurately, which means the testing period requires:
- 12 weeks of ad spend data
- At least 100 installs or events per day on average (depending on the target variable you choose to test).
3. Next, the marketer works to eliminate as many variables as possible. Any ongoing campaigns that were part of the analysis period are fine, but it’s important to avoid introducing any new channels, campaign formats, or actions that were not recorded in that 12-week period before analysis.
This means, for the seven days before and after the incrementality test, do NOT:
- Start any new campaigns or marketing actions.
- Changing the budget of ongoing marketing campaigns.
Then, incrementality analysis can only occur once seven days have passed from the initial trigger of the marketing action. This is so that the model has enough data to precisely predict what the outcome would have been without the marketing action.
In the case of Adjust’s InSight incrementality model, patterns in installs or events are recognized with input data like:
- App store ranking
- Ad spend
- Installs
- Monthly active users***
- Industry trends and significant external factors
- Daily sessions***
***These data points will be available in the next phase of InSight. Stay tuned!
The result? A clear and straightforward indication that your marketing action either caused:
- Incremental lift (more installs or events were conclusively due to a particular marketing action).
- Organic cannibalization (the increase of installs or events was only due to stealing it from organics because they were going to happen anyway) or statistically significant loss as a result.
- Or, no incremental value (no significant movement, positive or negative, detected).
Incrementality in action
Real-world results with InSight
Sleep Cycle, a leading sleep tracker app, was one of the first apps to harness InSight to refine and scale its marketing strategies without cannibalizing its strong organic traffic. Despite challenges posed by Apple's SKAdNetwork (SKAN) and the need for precise insights for product innovation and hyperlocalization, Adjust provided Sleep Cycle with the tools to measure the true impact of marketing activities.
Through Adjust's innovative approach, including the creation of synthetic control groups to navigate the post-device ID era, Sleep Cycle gained critical insights. This enabled them to experiment with new channels, optimize campaigns, and make data-driven decisions to avoid organic cannibalization and maximize investment in effective marketing channels.
Linnéa Gosh
Digital Marketing Analyst, Sleep Cycle
Consequently, Sleep Cycle has strengthened its market position, optimized its marketing spend, and maintained privacy-conscious practices, ensuring sustainable growth and enhanced marketing efficiency.
Adjust client iPocket Games also saw success with its early access to InSight.
Sakura Hong
Marketing Director, Hong Kong iPocket Technology Ltd
Ready, set, benchmark
Benchmarks from clients with early access to InSight
- 50% of channels tested showed cannibalization
- Most cannibalization came from gaming and e-commerce verticals
Benefits
The benefits of using incrementality analysis
As the industry moves away from granular user-level data towards a more aggregated methodology of measuring marketing efforts, marketers’ ability to surface details in real-time and understand the true impact of their efforts naturally is further complicated and hindered.
Yet, more is being asked of them. More precision, more agility, more efficiency, and more results. Adding incrementality analysis to a marketer’s tool belt can be game-changing in this complex landscape, as it provides a number of impactful benefits.
Budget agility
Confirming what’s lifting performance and what’s not with 95% precision makes it easy to optimize budget spending and changes as frequently and swiftly as needed for the highest performance.
Immunity against industry changes
Since incrementality is privacy-centric and data-aggregation-based, the incremental impact of campaigns can be measured as national and global privacy changes continue to roll out.
Resources and time reduction
Without the need for a dedicated data science team to comb through data on a daily basis, those resources can be freed up to focus on other matters. Better yet? Not having to invest the money in an in-house incrementality analysis or 3rd-party solution while still getting valuable insights about campaign performance right from the comfort of the Adjust dashboard.
Confidence and support to innovate
Having a precise way to validate new channels, ad formats, countries, seasonality, and other alternative marketing tactics allows marketers to confidently leave their comfort zone to expand their reach and audience and diversify marketing strategies.
Partner accountability
With more visibility into organic cannibalization, marketers can have honest conversations with ad networks when it comes to making the necessary optimizations to achieve success with them.
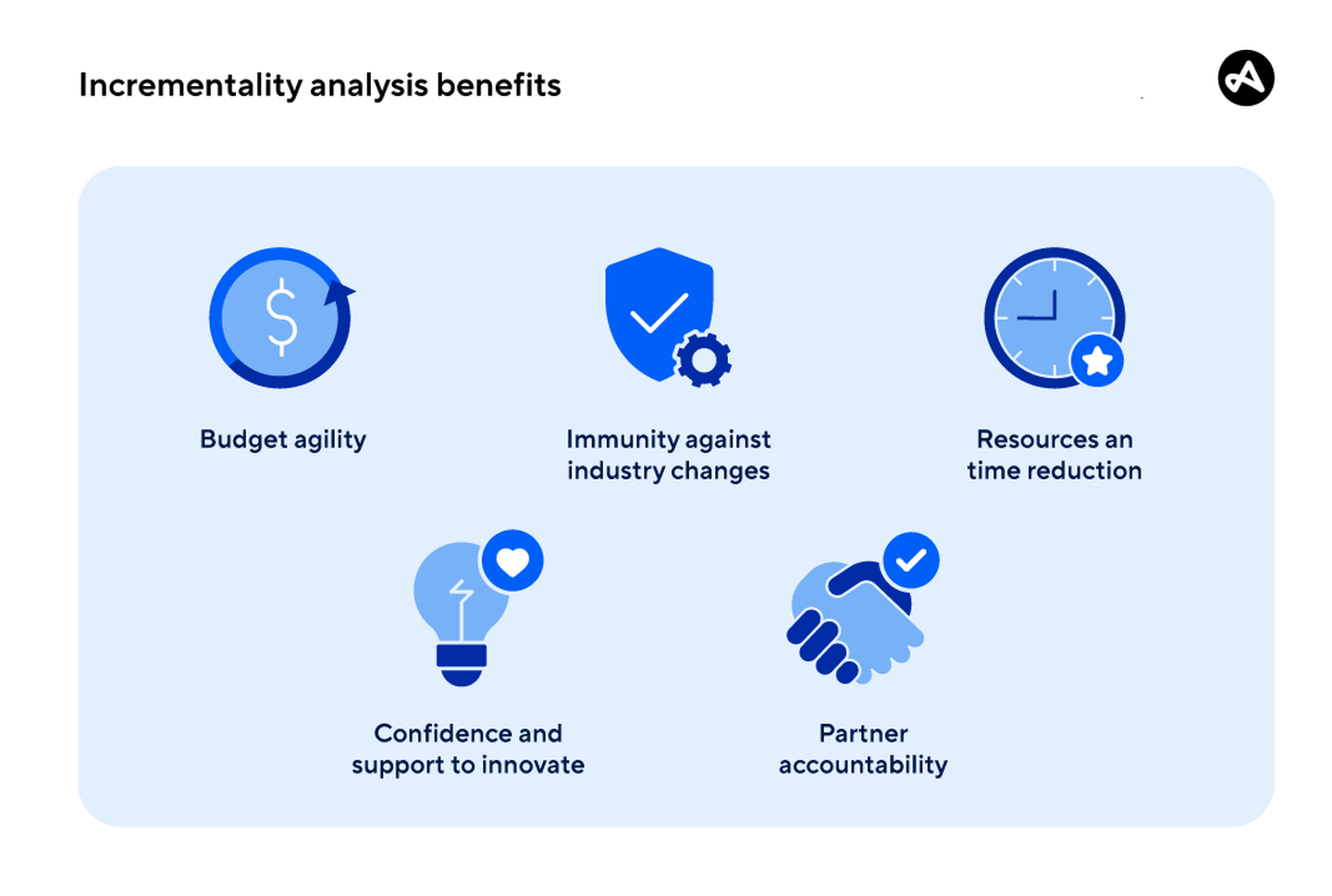
Best practices
Best practices for optimal incrementality testing
Let’s run through a few tips and tricks to get the most out of your incrementality analysis.
-
Start with a clear hypothesis.
Be as specific as possible about the outcome you were hoping to get. For example, if you’ve decided to double your budget with network A, your hypothesis might be a 10% increase in Android installs in China.
-
Test campaigns with identified impact.
Because you’ll have historical data, make sure to first identify a causal relationship between your campaign and KPI. Then, you’ll be able to measure this impact with incrementality analysis. This means that if your campaign didn’t move the needle, then it’s not worth testing for incremental value.
-
Know how to preserve data reliability.
If you’re already an Adjust client, we’ve got you covered! Any activity for which we already have ad spend data can be fed into our models. If you’re running marketing campaigns that don’t automatically have ad spend measured by us (either offline, on your own channels, via affiliates, etc.), then you must make sure that Adjust is getting the ad spend information. Or, avoiding running those particular campaigns during the pre- and post-analysis periods.
As we continue to navigate the complexities of today’s marketing landscape, marked by stringent data privacy regulations and rising user acquisition costs, the necessity for precision and agility in marketing strategies has never been more critical. Incrementality analysis emerges as a powerful tool in this context, offering marketers a clear, data-driven lens through which to evaluate the effectiveness of their campaigns beyond the confines of traditional metrics.
Adjust's innovative approach to incrementality, through our InSight solution, underscores the importance of adaptability and precision, enabling marketers to uncover the true impact of their efforts, optimize their strategies in real-time, and confidently drive sustainable growth. By embracing the best practices for incrementality testing and leveraging Adjust’s sophisticated machine learning algorithm, marketers can ensure that their campaigns are not just data-compliant but poised for maximum impact, steering their brands towards greater efficiency and effectiveness in an ever-evolving digital ecosystem.
Ready to get started with Adjust InSight today? Request a demo and we’ll show you how to take your marketing measurement to the next level with incrementality analysis.
Want to get the latest from Adjust?